Harnessing the benefits of Large Language Models (LLM)
DeepKeep enables enterprises to harness the benefits of LLM while avoiding the risks associated with using them
Deepkeep’s solution for LLM
Security and trustworthiness are critical not only when using an external LLM (like ChatGPT, Claude, or Bard) for content generation, summaries, translations, etc., but even more so when using internal fine-tuned LLM.
Protect against LLM attacks, including prompt injection, adversarial manipulation and semantic attacks
Identify and alert against hallucination using a hierarchical system of data sources, including both internal and trusted external references
Safeguard against data leakage, protecting sensitive data and personally identifiable information (PII)
Detect and removes toxic, offensive, harmful, unfair, unethical, or discriminatory language
Security
Securing AI models from the development phase of machine learning models through the entire product lifecycle, including risk assessment, protection, monitoring and mitigation.
Poisoning
When hackers purposely change a model or dataset.
Denial of Service
When attackers consume excessive resources while interacting with an LLM.
Evasion
When attackers mislead an LLM to produce incorrect or biased predictions.
Data leakage
When an LLM reveals confidential, sensitive or proprietary information through its responses.
PII Privacy
When a model jeopardizes personal identifiable information, such as digits that are part of a social security number, or bank account.
Trustworthiness
Valid, reliable, resilient, accountable, fair, transparent and explainable.
Fairness
When an LLM discriminates based on gender, age, ethnicity, or anything else.
Toxicity
When an LLM is rude, disrespectful, or makes an unreasonable comment. F*cking unbelievable!
Hallucination
When a model’s response contains inaccurate information, producing outputs that are nonsensical, misleading, or irrelevant.
LLMs lie and make mistakes. The question is not if, but when and how.
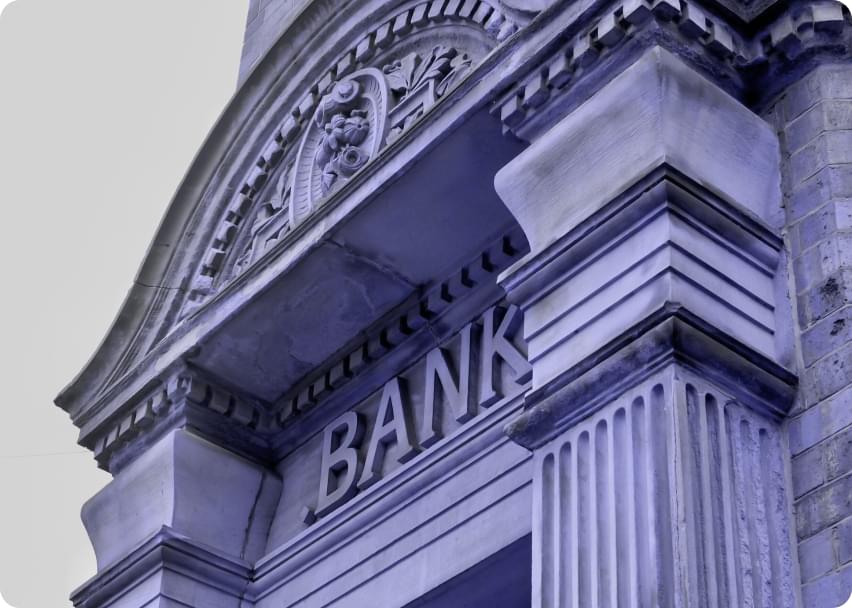
Security Breach
Banks are already using AI models to streamline decision-making processes about a wide rangeof topics, such as credit and algorithmic trading. However, AI models are subject to anomalousand malicious inputs, as well as unexpected behavior.
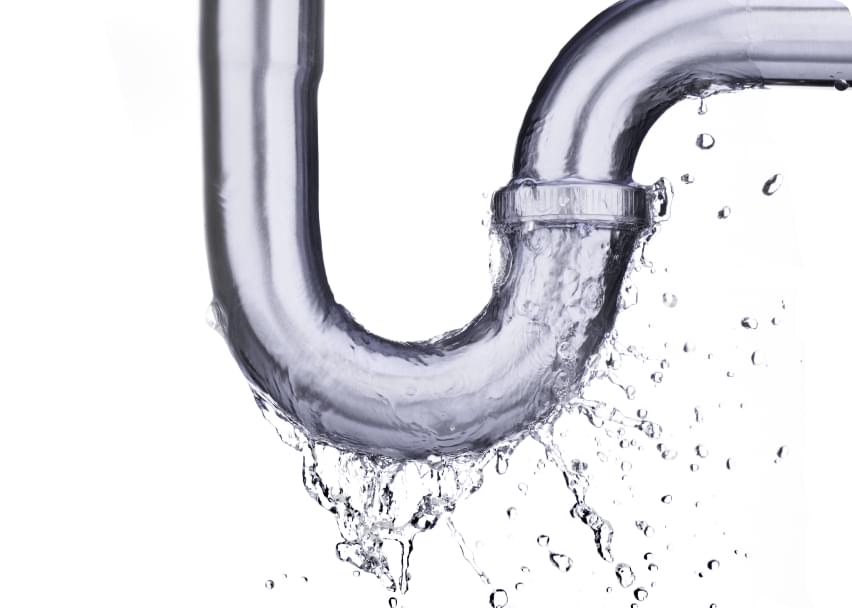
Exploiting Data Leakage
An insurance company employs LLM-based customer support by utilizing a walledgarden application. This application has been trained to steer away from any questions notpertaining to predetermined topics. However, attackers are exploiting customer supportinterfaces, breaching LLM guardrails to extract data that goes beyond the intended scope ofassistance.